Understanding **Bounding Boxes** in Data Annotation
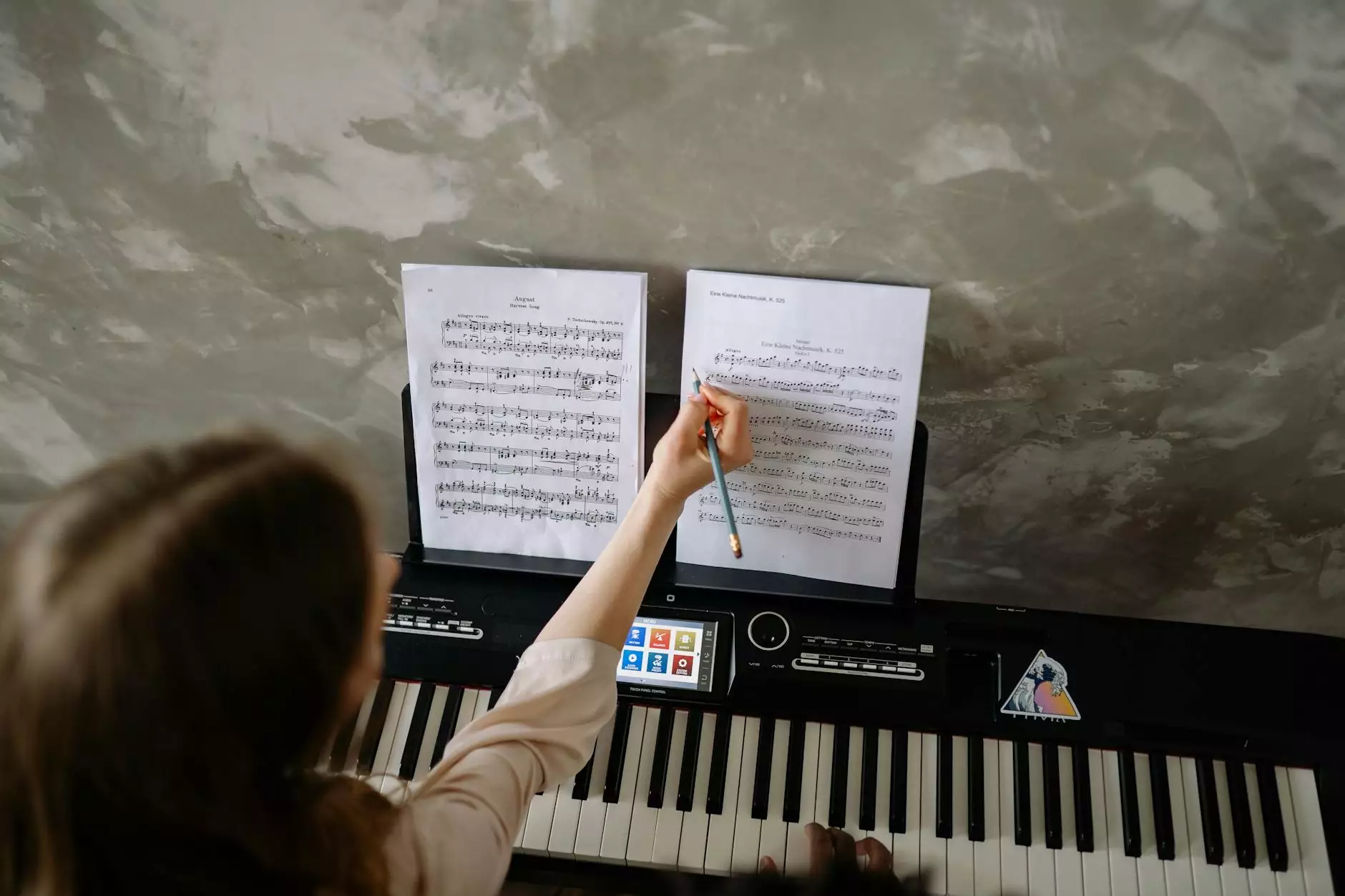
In the rapidly evolving world of artificial intelligence (AI) and machine learning (ML), the significance of accurate data annotation cannot be overstated. Among the many tools and techniques used in this domain, bounding boxes occupy a crucial position. This article aims to explore the concept of bounding boxes thoroughly, their applications, and how they play a significant role in enhancing the efficacy of data annotation tools and platforms.
What are Bounding Boxes?
Bounding boxes are rectangular frames used to highlight and describe objects within images or videos. They serve as a fundamental tool in the process of data annotation, allowing algorithms to identify and classify objects effectively. In the context of machine learning, bounding boxes are particularly vital because they enable models to understand the spatial and contextual relationships between different objects.
The Importance of Bounding Boxes in Data Annotation
In data annotation, bounding boxes play several essential roles, particularly in the following areas:
- Object Detection: Bounding boxes are indispensable for training models to recognize and locate objects within visuals. Without them, AI systems may struggle to identify where one object ends, and another begins.
- Image Segmentation: While bounding boxes provide a straightforward method to annotate objects, they can also be a precursor to more advanced techniques like image segmentation, which involves delineating objects with greater precision.
- Improving Model Accuracy: Properly annotated data, including well-placed bounding boxes, enhances the predictive power of machine learning models, resulting in improved accuracy in real-world applications.
How to Create Effective Bounding Boxes
Creating effective bounding boxes involves several key considerations:
1. Precision in Drawing
The accuracy of the bounding box is paramount. It should closely follow the edges of the object being annotated. Inaccurate placement or size can lead to poor model performance. Utilizing advanced annotation tools can assist in achieving precision.
2. Consistency Across Annotations
When annotating a dataset, consistency is crucial. Using the same labeling criteria for all images ensures that the model receives uniform training data, which is vital for its learning process. Documenting guidelines for annotators can help maintain this consistency.
3. Handling Overlaps
In cases where multiple objects overlap, carefully distinguishing between them with appropriate bounding boxes is essential. Annotators must take care to avoid encasing multiple objects in one bounding box, which could confuse the model during training.
The Role of Bounding Boxes in Different Industries
Bounding boxes find applications across various sectors, showcasing their versatility and importance. Here are a few notable examples:
1. Autonomous Vehicles
In the automotive industry, self-driving cars rely on precise bounding boxes to detect pedestrians, traffic signals, road signs, and other vehicles. The reliable detection of these objects is critical for safe navigation.
2. Retail and E-Commerce
In the retail sector, companies use bounding boxes in their visual search functionalities. By annotating product images, AI systems can recommend similar items, enhancing the shopping experience for customers.
3. Healthcare
In medical imaging, bounding boxes are used to identify specific areas of interest, such as tumors in X-rays or MRIs. Correctly annotated images can significantly aid radiologists and medical professionals in making accurate diagnoses.
Advanced Techniques in Using Bounding Boxes
As technology progresses, the methods for using bounding boxes are also evolving. Here are some advanced techniques that harness the power of bounding boxes:
1. Integration with Deep Learning Models
Modern deep learning frameworks utilize bounding boxes as inputs for training convolutional neural networks (CNNs). These models learn to predict the presence and location of objects based on annotated bounding boxes, ultimately improving their accuracy in object detection tasks.
2. Augmentation of Training Data
Bounding boxes also support data augmentation techniques that enhance the diversity of training data. By manipulating the bounding boxes within images—such as rotating, flipping, or scaling—they can create more training scenarios for models, improving their robustness.
3. Transition to Semantic Segmentation
Bounding boxes are often the first step before moving to semantic segmentation. While bounding boxes provide a coarse understanding of an image, semantic segmentation can produce pixel-level annotations, allowing for even finer distinctions between object classes.
Challenges Involved with Bounding Boxes
Despite their usefulness, working with bounding boxes does present certain challenges:
1. Annotator Bias
Human annotators may introduce bias through subjectivity in their placements of bounding boxes. Providing clear guidelines and training can mitigate this issue, ensuring a more consistent annotation process.
2. Handling Complex Objects
Complex objects with irregular shapes might not fit neatly within a rectangular bounding box. In such cases, it may be more appropriate to utilize polygonal annotations for better accuracy.
3. Time-Consuming Process
The manual creation of bounding boxes can be labor-intensive and time-consuming. Investing in automatic annotation tools with AI-assisted capabilities can significantly speed up the process, reducing the need for extensive human intervention.
Choosing the Right Data Annotation Platform for Bounding Boxes
For businesses looking to implement effective bounding boxes in their data annotation strategy, selecting the right platform is crucial. Here are some key characteristics to consider:
- User-Friendly Interface: The platform should offer an intuitive interface that allows annotators to work efficiently without extensive training.
- Support for Multiple Formats: A flexible platform can accommodate various data formats (images, videos) and object types for comprehensive annotation capabilities.
- Collaboration Features: The ability for multiple users to collaborate in real-time can enhance productivity and ensure quality control.
- Integration Capabilities: Look for platforms that seamlessly integrate with existing workflows and machine learning pipelines.
Conclusion: The Future of Bounding Boxes in AI
As AI continues to advance, the role of bounding boxes in data annotation will likely grow even more prominent. Their ability to simplify object detection and improve machine learning accuracy makes them indispensable tools in the data annotator’s toolkit. Platforms like keylabs.ai are at the forefront of this transformation, providing robust data annotation solutions that leverage the power of bounding boxes to meet the demands of evolving technologies.
By understanding and effectively utilizing bounding boxes, businesses can ensure their AI models are trained on high-quality data, paving the way for groundbreaking applications across various industries. The future holds exciting possibilities as we continue to refine our methodologies and embrace advanced technologies in the realm of data annotation.