The Importance and Impact of Agriculture Datasets for Machine Learning
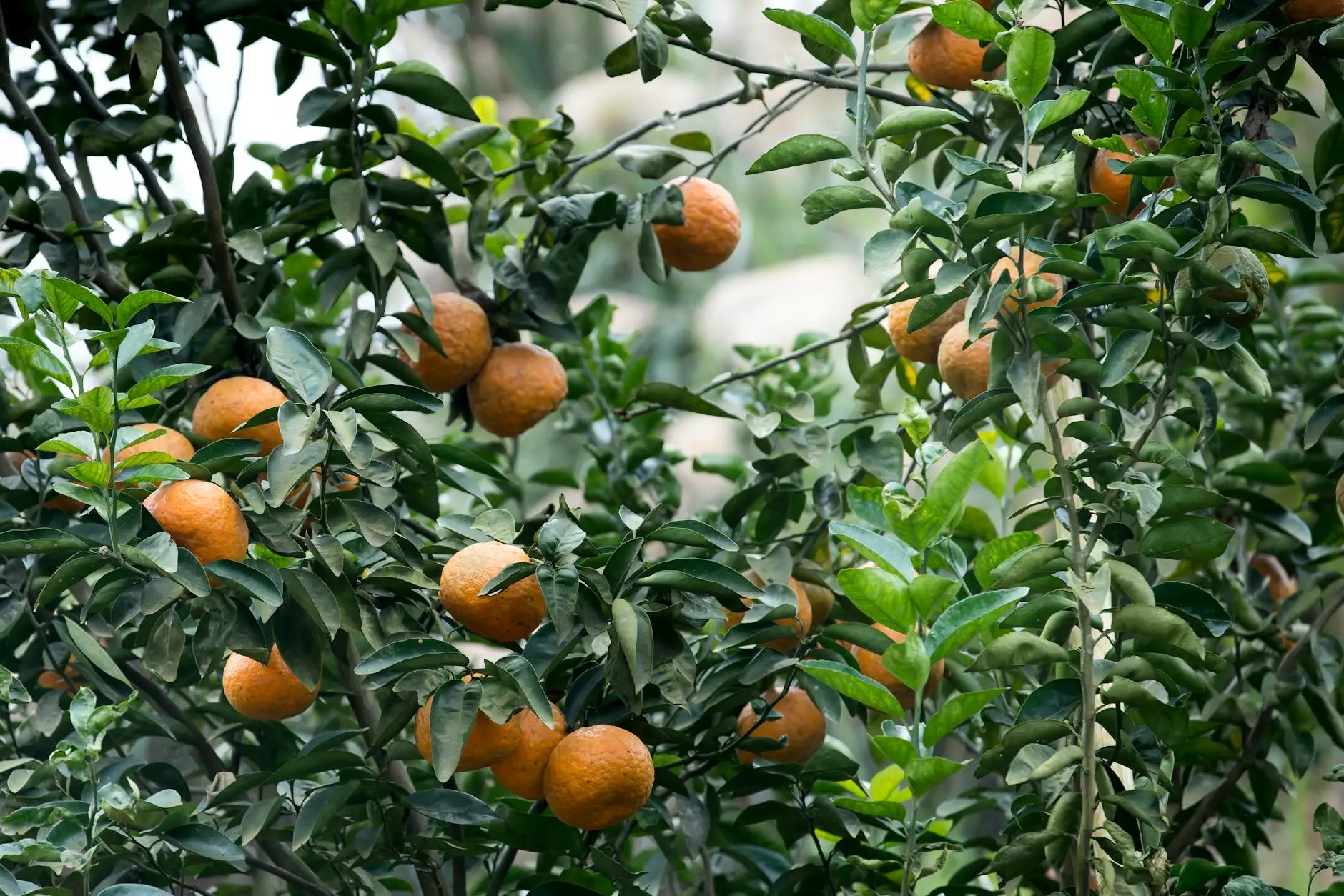
In the era of digital transformation, the agricultural sector has not been left behind. Among the most profound influences on this industry is the utilization of agriculture datasets for machine learning. These datasets serve a crucial role in advancing agricultural practices, improving yield, and ensuring sustainability. This article delves into what agriculture datasets are, their applications in machine learning, and how they are revolutionizing the farming landscape.
Understanding Agriculture Datasets
Agriculture datasets are collections of data specifically related to the cultivation of crops and livestock. They can encompass various data types, such as:
- Weather Data: Information regarding temperature, rainfall, humidity, and other atmospheric conditions.
- Soil Data: Properties of soil types, pH levels, nutrient content, and soil moisture levels.
- Crop Yield Data: Historical yield data for various crops which helps ascertain trends and forecasts.
- Market Data: Prices, demand and supply indexes, and other economic factors affecting agriculture.
- Pest and Disease Ratings: Information on pest outbreaks and disease prevalence that can affect crop health.
These datasets are often collected from a variety of sources, including agricultural research organizations, government databases, and private sector companies. When compiled, they become powerful tools for data analysis and decision-making in agriculture.
The Role of Machine Learning in Agriculture
Machine learning (ML) enables systems to learn from data, improving their performance through experience. In agriculture, ML algorithms analyze vast amounts of data, offering insights that were previously unattainable. Here are some ways machine learning is utilized in agriculture:
1. Crop Prediction and Management
One of the most significant applications of agriculture datasets for machine learning is in predicting crop yields. By analyzing historical data, machine learning models can forecast how different factors like weather conditions, soil quality, and pest infestations will affect crop production.
These predictive models allow farmers to make informed decisions regarding:
- Which crops to plant based on market demand and historical performance.
- Optimal planting times to maximize yield.
- Efficient resource allocation, such as water and fertilizers.
2. Precision Agriculture
Precision agriculture employs machine learning algorithms to optimize field-level management regarding crop farming. By leveraging agriculture datasets with geographical information, farmers can apply the right amount of resources in the right places, thereby reducing waste and enhancing productivity.
Examples of precision agriculture practices include:
- Variable Rate Technology (VRT): Adjusting the application rates of inputs such as fertilizers and pesticides based on data-driven insights.
- Remote Sensing: Utilizing drones and satellite imagery to gather real-time data about crop health and soil conditions.
3. Disease and Pest Management
Predictive analytics powered by machine learning can help identify potential disease outbreaks and pest infestations before they spread significantly. By analyzing patterns from agriculture datasets, farmers can take preventive measures—targeting specific areas with pesticides or applying disease-resistant crop varieties.
Benefits of Using Agriculture Datasets for Machine Learning
The integration of agriculture datasets for machine learning offers numerous advantages:
1. Enhanced Decision-Making
With data-driven insights, farmers can make better decisions that lead to improved outcomes. Whether it’s optimizing planting schedules or calculating the best timing for herbicide applications, these insights can result in substantial cost savings and increased yields.
2. Increased Efficiency and Productivity
Machine learning helps in identifying inefficiencies in existing practices. For example, by determining the most effective irrigation methods or understanding when to harvest, farmers can boost their productivity significantly.
3. Sustainability
As the world confronts climate change and resource scarcity, sustainable practices are crucial. Machine learning can guide sustainable farming practices by optimizing resource use and reducing environmental impact.
Challenges in Implementing Machine Learning in Agriculture
Despite the benefits, the deployment of machine learning in agriculture is not without challenges:
1. Data Quality and Accessibility
The effectiveness of machine learning models relies heavily on the quality of the data being used. Inconsistent data quality across various sources can yield unreliable outcomes. Moreover, not all farmers have access to comprehensive datasets, which can limit the widespread adoption of these technologies.
2. Complexity of Models
Machine learning models can become complex and challenging to interpret. Farmers, especially those without technical backgrounds, may find it difficult to trust or utilize recommendations derived from sophisticated algorithms.
3. Cost of Technology
Investing in the technology needed to gather data—from sensors to drones—and the software for analysis can be prohibitively expensive for many small-scale farmers.
Future Trends in Agriculture Datasets and Machine Learning
As the intersection of agriculture and technology evolves, several trends are emerging that promise to shape the future of farming:
1. Integration of IoT Devices
The Internet of Things (IoT) is poised to revolutionize agriculture by providing real-time data through sensors connected to the internet. This data can significantly enhance the datasets available for machine learning, allowing for more precise analytics and insights.
2. Improved Machine Learning Algorithms
As technology progresses, machine learning algorithms will become more sophisticated and capable of processing larger datasets with greater accuracy. This will facilitate even more advanced applications in agriculture, such as autonomous farming techniques.
3. Collaborative Data Sharing
Future advancements are likely to emphasize collaborative data sharing among farmers, researchers, and organizations. By pooling resources and datasets, the agricultural community can develop more comprehensive insights and foster innovation collectively.
Conclusion
In summary, the integration of agriculture datasets for machine learning is transforming the agricultural industry by enhancing productivity, sustainability, and decision-making. As challenges regarding data accessibility and technology costs are addressed, the potential for these advanced technologies to reshape farming practices will continue to expand. For businesses looking to succeed in this new landscape, embracing data-driven strategies will be essential to staying competitive and resilient in an ever-changing world.
Call to Action
For both large-scale agricultural operations and small family farms, now is the time to explore the potential of agriculture datasets and machine learning. Invest in the tools and technologies that allow for data collection and analysis, and reap the benefits of informed decision-making. For more insightful resources on how to leverage technology in agriculture, visit keymakr.com.
agriculture dataset for machine learning