Unlocking the Power of Image Datasets for Object Detection
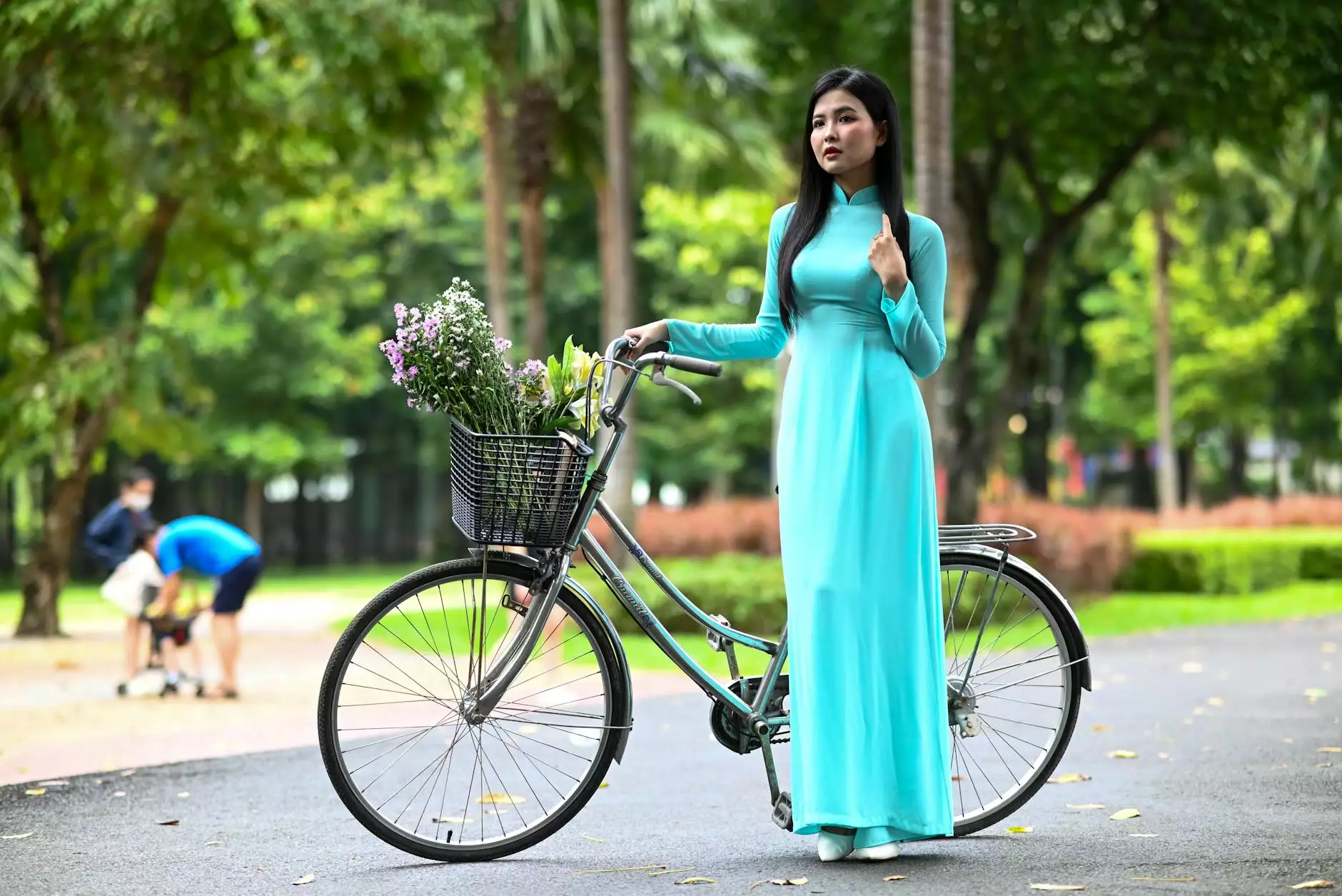
In today’s digital landscape, businesses are increasingly leveraging image datasets for object detection to enhance their machine learning capabilities. Whether it’s for self-driving cars, computer vision applications, or security systems, the demand for efficient and accurate object detection is skyrocketing. This article delves deep into the essentials of image datasets, the role of data annotation tools, platforms, and how keylabs.ai stands at the forefront of this technological revolution.
What is Object Detection?
Object detection is a pivotal task in computer vision that involves identifying and locating objects within an image. This technology is not merely about recognizing objects but also entails determining their position within an image through bounding boxes. Applications of object detection span various industries:
- Automotive: Enhancing driver assistance systems and enabling autonomy in vehicles.
- Healthcare: Assisting in diagnostics through medical imagery analysis.
- Retail: Facilitating inventory management and customer behavior analysis through surveillance.
- Security: Identifying threats in real-time by analyzing surveillance footage.
The Importance of Image Datasets in Object Detection
At the heart of effective object detection lies the image dataset. These datasets comprise a large collection of annotated images, which provide the essential fuel for training machine learning models. The quality and quantity of data available can significantly affect the performance of an object detection algorithm. Here are several reasons why image datasets for object detection are crucial.
1. Variety and Diversity
Effective object detection systems demand diverse datasets that include various orientations, lighting conditions, backgrounds, and object scales. This diversity ensures that the model can generalize well to new, unseen data. For instance, datasets used in training may include images of cars taken from different angles, in various weather conditions, and set against different backdrops.
2. Accuracy
High-quality annotated datasets play a vital role in improving detection accuracy. Annotations guide the machine learning model in learning from both positive and negative examples. Poorly annotated datasets can lead to misclassified objects and a higher rate of false positives. Therefore, ensuring the perfection of data annotation is paramount, which is where platforms like keylabs.ai excel.
3. Capacity for Training Deep Learning Models
Deep learning models, particularly Convolutional Neural Networks (CNNs), require extensive training data to achieve optimal performance. The more diverse and comprehensive the dataset, the more capable the model becomes in identifying and classifying objects within images. This reliance on rich image datasets enhances a model's ability to learn complex patterns effectively.
Data Annotation: The Backbone of Object Detection
Effective data annotation transforms raw images into meaningful datasets that fuel machine learning algorithms. This process involves various forms of labeling, including:
- Bounding Boxes: Drawing rectangles around targeted objects.
- Segmentation Masks: Outlining the exact shape of objects for more accuracy.
- Keypoints: Identifying specific points of interest within an object (such as facial features).
- Class Labels: Assigning categories to recognized objects.
The choice of annotation type directly impacts the performance of the object detection model. For instance, bounding boxes provide a faster annotation method, but segmentation masks allow for finer discrimination of object shapes. As a result, selecting the right data annotation tool is critical for businesses aiming to make the most of their image datasets.
Introducing keylabs.ai: A Premier Data Annotation Platform
keylabs.ai is revolutionizing the landscape of data annotation with its advanced tools and platforms tailored for image datasets for object detection. Our innovative solutions ensure that businesses receive high-quality annotated datasets that meet their specific needs. Here’s what sets keylabs.ai apart from the competition:
1. Cutting-edge Technology
Utilizing the latest advancements in artificial intelligence and machine learning, keylabs.ai automates various facets of the data annotation process. This not only speeds up the annotation cycle but also enhances the accuracy of the annotations, allowing for more reliable training of object detection models.
2. Human Expertise
While technology plays a significant role, human oversight remains critical. At keylabs.ai, we employ a team of expert annotators who ensure that every image annotation meets the highest standards. This blend of technology and human expertise guarantees precision and quality, resulting in datasets that clients can trust.
3. Scalability
As businesses grow, so do their data requirements. Our platform offers scalable solutions that adapt to the size and complexity of your object detection projects. Whether working with a few hundred images or several million, keylabs.ai can handle it all seamlessly.
Case Studies: Success Stories with Image Datasets
Learning from others' success is invaluable in the tech industry. Here are a few case studies demonstrating how companies have effectively utilized image datasets for object detection through keylabs.ai:
1. Autonomous Vehicle Development
A leading automotive manufacturer partnered with keylabs.ai to develop an object detection system for their autonomous vehicles. Using tailored image datasets annotated for diverse driving scenarios, they achieved remarkable improvements in their vehicles' ability to recognize pedestrians, signs, and other vehicles, boosting safety and compliance with regulations.
2. Retail Analytics
A major retail chain leveraged keylabs.ai to analyze customer behavior through video surveillance. The annotated datasets helped them detect foot traffic patterns and customer interactions with products, allowing for tailored marketing strategies and enhanced in-store experiences.
3. Medical Imaging Enhancements
In the healthcare sector, a diagnostics company employed keylabs.ai to annotate medical images with a focus on disease detection. The precise annotations led to improved algorithms for identifying tumors and other abnormalities, significantly enhancing diagnostic accuracy.
Best Practices for Creating Image Datasets
To achieve optimal results in object detection, businesses should adhere to the following best practices when creating or utilizing image datasets for object detection:
1. Ensure Comprehensive Annotation
The dataset should cover an extensive range of scenarios and variations. It’s vital to label objects accurately and comprehensively to capture all possible appearances and perspectives of the items being classified.
2. Utilize multiple Image Sources
Incorporate images from various sources to create a more diverse dataset. This diversity improves the model’s performance across different contexts and reduces the risk of overfitting to specific conditions.
3. Regular Updates
Data relevancy degrades over time, especially as new objects or categories emerge. Regularly updating datasets and annotations is crucial to maintaining accuracy and effectiveness in object detection models.
The Future of Object Detection
As technology progresses, the future of object detection looks even more promising. The integration of artificial intelligence with image datasets will yield more intelligent systems capable of real-time decision-making and predictive analysis. Here are some anticipated trends:
- Enhanced Automation: Future annotation tools will be even more automated, reducing human error and expediting the annotation process.
- Integration with Augmented Reality: Object detection systems will likely integrate with AR technologies to deliver enhanced user experiences across various applications.
- Advancements in 3D Object Detection: Future datasets may focus on 3D spatial representations, allowing for even greater accuracy in recognizing and classifying objects in complex environments.
Conclusion
In conclusion, the significance of image datasets for object detection cannot be overstated. They form the backbone of effective machine learning models, powering innovations across various sectors. As businesses strive to harness the true potential of object detection, utilizing a reliable and advanced platform like keylabs.ai will be crucial.
By focusing on high-quality data annotation, comprehensive datasets, and cutting-edge technology, organizations can pave the way for more accurate and efficient object detection systems. Always keep in mind that the quality of your dataset is directly proportional to your machine learning model’s potential — invest wisely!
image dataset for object detection